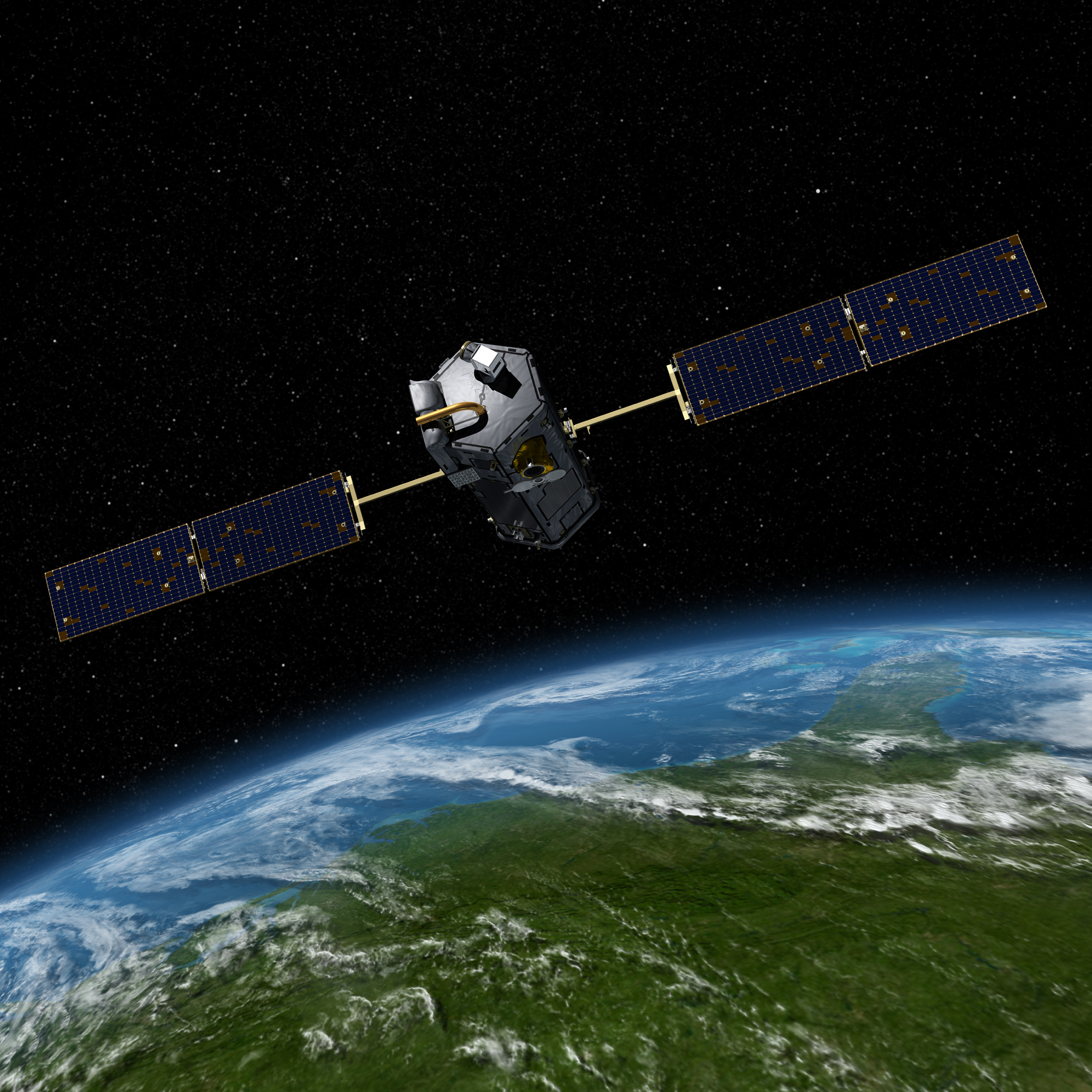
The Uncertainty Quantification and Statistical Analysis Group carries out research and technology development in computational techniques to extract knowledge from science data.
What we do: Model the statistical variability of physical systems like oceans and atmospheres; infer parameters, recognize patterns, and quantify uncertainty in massive data sets taken from such systems.
Uncertainty Quantification
- Uncertainty quantification (UQ) for retrievals from Earth-observing instruments including OCO-2, MLS, ECOSTRESS, and MAIA
- Uncertainty assessment and propagation for a large-scale hydrological routing model, and uncertainty assessment for groundwater using models and gravimetric observations
Statistical Data Fusion and Science Data Analysis
- Spatial and spatio-temporal data fusion for atmospheric fields in a Bayesian context, with applications to near surface temperature products from AIRS
- Developed a leading system for recognizing, grouping, and tracking solar active regions used for SOHO and distributed as a data product for SDO
- Proprioceptive and appearance-based terrain classification for autonomous rover navigation (DARPA, ARO)
- Developed multi-observation compressed summaries for MISR and AIRS, which have been distributed as a Level 3 data product
- Developing new statistical methods for assessing agreement between climate model simulations and observations in a distributed data context
Physical Modeling and Advanced Inversion Algorithms
- Inversion of cosmic microwave background to recover spherical harmonic power, and structural parameters of cosmological models (Wilkinson Microwave Anisotropy Probe and Planck)
- Data assimilation and Bayesian risk assessment for highly nonlinear weather systems
A 2019 seminar highlighted recent activities in data fusion and uncertainty quantification:
-
Hai Nguyen
“Spatial Statistical Data Fusion for Remote Sensing Applications” (CL#19-7476)
Spatial statistical methods for combination of measurements from multiple instruments, including bias removal. -
Amy Braverman
“Uncertainty Quantification for Science and Engineering Applications” (CL#19-7610)
Overview of uncertainty quantification in science and engineering domains.
-
Vineet Yadav
“Real-time Modeling and Software Framework for Estimating Greenhouse Gas Emissions” [with movies: PowerPoint version] (CL#15-5676)
Moving from CO2 concentrations to CO2 emissions using spatio-temporal transport models -
Jonathan Hobbs
“Uncertainty Quantification for Remote Sensing Retrievals: Monte Carlo Experiments for OCO-2” (CL#15-5287)
Theory and practice for computing well-justified error bars for OCO-2 retrievals -
Fred Calef
“Science Data Localization and Access via webGIS: MSL Test Case”
Putting all geo-located Mars data into an interactive interface for high-tempo scientifically-informed operations